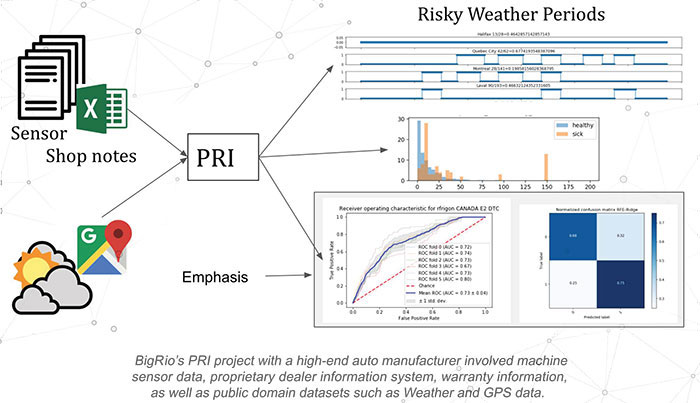
A far more ideal method for fleet management is what’s known as predictive maintenance, whereby an intelligent algorithm takes in numerous measurements and predicts the pending component failure with a high degree of accuracy. From hereon, we refer to this algorithm as Predictive Repair Intelligence or PRI. In general, predictive models of this sort work either by classification for a breakdown within a prediction horizon, or by means of a Remaining Useful Life (RUL) assessment. While the application of supervised learning to such a problem can seem mundane, dealing with a real-life PRI case proves to be anything but straightforward. Here, in the rubber meets the road scenario, academic clarity counts much less than hard knuckled experience with real data, domain folklores, and the wild west of IoT sensors.
In a typical Data Science engagement, our team of experts walks into a hardware centric environment, where the client staff are of a deterministic logic mindset, with little prior exposure to statistical ways of problem solving. Design experts debug component failures by diving deeply into individual cases, juggling up to 4 or 5 failure instances maximum in his head. While information exchange is both interesting and informative, domain guidance alone rarely directly yields a workable set of relevant input signals and reliable labeled samples.